Designing for AI: Bridging User Experience and Machine Learning
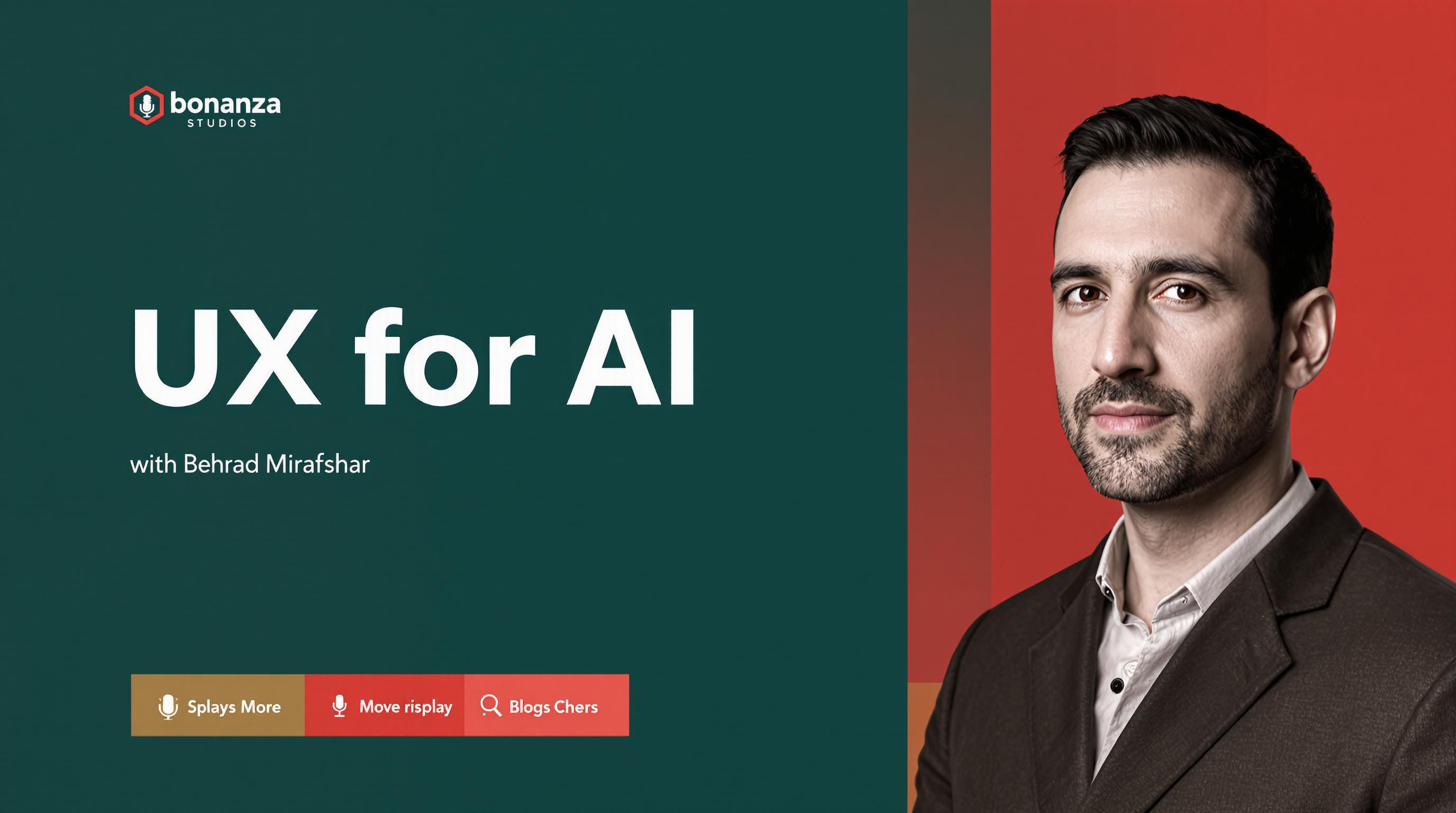
AI products succeed when they’re designed with users in mind. To build trust and ensure adoption, UX designers focus on three key areas:
- Personalization: Tailor experiences like Spotify’s playlists or Duolingo’s adaptive lessons.
- Transparency: Explain AI decisions, as seen in LinkedIn’s “Explain Your Match” or Grammarly’s confidence indicators.
- User Control: Allow adjustments, such as Tesla’s customizable Autopilot settings.
Creating ethical AI also means respecting user rights, ensuring compliance with laws like GDPR, and addressing biases through diverse testing and data practices. Tools like FairNow and Enzai help organizations stay compliant, while AI-driven design tools simplify building intuitive, accessible interfaces.
Designing For AI: Principles, Frameworks, and Practical Insights
How AI Changes UX Design
Integrating AI into UX design aims to make technology both user-focused and easy to understand. This involves strategies like personalization, clear decision-making, and ongoing testing. These approaches address the challenges of blending AI with everyday user interactions.
Personalizing User Experiences with AI
Today, 71% of U.S. consumers expect tailored interactions. Companies like Starbucks, Spotify, and Duolingo showcase how AI meets this demand:
- Starbucks uses location data to recommend nearby stores.
- Spotify creates playlists based on the time of day and user preferences.
- Duolingo adjusts lessons based on individual progress.
These personalized experiences can drive up to 40% more revenue for businesses.
Explaining AI Decisions to Build Trust
Personalization is important, but making AI decisions understandable is just as critical for maintaining user trust. UX designers now incorporate tools like visual decision trees, detailed explanations, and interactive feedback to clarify AI processes.
For example:
- LinkedIn uses its "Explain Your Match" feature to show why certain jobs are recommended, based on user data.
- Grammarly adds confidence indicators to its writing suggestions, empowering users to make better-informed decisions.
Testing AI with Real Users
Testing AI systems involves evaluating accuracy, gathering user feedback, and ensuring the system aligns with ethical standards. For instance, Tesla's Autopilot allows users to adjust assistance levels, maintaining a balance between automation and user control. These testing practices help refine AI behavior to better meet user expectations.
Building Ethical AI Products
Designing AI products ethically means focusing on user rights, compliance with laws, and creating accessible interfaces. Prioritizing these elements helps ensure privacy and fosters trust.
Incorporating User Rights into Design
Ethical AI begins with mapping user interactions while respecting fundamental rights. Here are some critical factors to consider:
- Data transparency: Clearly explain how user data is collected, stored, and used.
- User control: Offer clear opt-in and opt-out options for AI features.
- Decision visibility: Make AI-driven recommendations and their effects easy to understand.
In addition to addressing user rights, aligning designs with legal requirements is equally important.
Designing for AI Regulations
Once user rights are addressed, meeting the requirements of AI regulations becomes a priority. Laws like the EU AI Act and GDPR demand careful attention during the design process. Effective approaches include:
- Regular compliance reviews throughout the design cycle.
- Conducting audits to identify and mitigate bias.
- Handling user data with care and sensitivity.
Improving AI Interfaces
Clear communication and empowering users can significantly reduce barriers in AI interfaces, complementing ethical design and legal compliance.
"Users need to understand AI to trust it." – Design Agency for AI startups
For example, Grammarly minimizes interface challenges by:
- Showing confidence levels for AI suggestions.
- Explaining the reasoning behind recommendations.
- Allowing users to make corrections instantly.
Similarly, Microsoft's Seeing AI app exemplifies ethical design by offering features like real-time descriptions of surroundings and text reading, making AI tools accessible to visually impaired users.
sbb-itb-e464e9c
Tools for AI Product Design
Developing AI products today requires specialized tools that simplify the process and help meet regulatory requirements. These tools combine compliance measures with cutting-edge design capabilities.
Tools for Legal Compliance in AI
As AI regulations become more complex, compliance tools are essential:
- FairNow: Monitors compliance continuously, sending alerts when regulations change to ensure transparent AI practices.
- Enzai: Automates compliance assessments, offering a clear view of an organization's AI regulatory status.
- EU AI Act Compliance Checker: Helps organizations understand their specific obligations under new regulations, breaking down complex requirements.
AI-Driven Design Tools
Many organizations are incorporating AI into their design processes. Companies like The New York Times and Walmart have even introduced roles like "Senior Design Editor, AI Initiatives" to lead these efforts.
Modern AI design tools offer features such as:
- Real-time interface updates based on user behavior.
- Automatic adjustments to improve accessibility.
- Multi-modal input and output systems for better user interaction.
"Organizations trust FairNow's AI governance platform to maximize their AI investments while strategically minimizing compliance-related risks." – FairNow
Incorporating Text Processing into Interfaces
Natural Language Processing (NLP) plays a key role in building effective AI interfaces. For example, Google Assistant uses contextual suggestions and visual prompts to guide users in discovering its features intuitively. Similarly, LinkedIn's "Explain Your Match" feature clarifies how job recommendations are made, boosting transparency.
To integrate NLP effectively, consider these approaches:
- Add confidence indicators to AI suggestions.
- Offer layered feedback, from simple summaries to detailed breakdowns.
- Develop interactive error recovery systems with alternative suggestions.
- Include clear opt-out options to give users control.
The best AI product design tools strike a balance between advanced functionality and user-friendly interfaces while keeping up with regulatory changes.
Solving Common AI Design Problems
Addressing challenges in AI design is vital for creating systems that are ethical, transparent, and easy to use. Building on earlier discussions about ethics and regulation, let’s explore practical ways to tackle common issues in AI design.
Setting Clear User Expectations
For AI systems to succeed, users need to understand how they work and feel in control. Transparent interfaces and clear communication play a big role in building trust. One effective method is using layered feedback systems. For instance, Grammarly’s interface provides confidence indicators alongside tailored suggestions, giving users the option to dive deeper into details if they choose. Similarly, Tesla’s Autopilot blends automation with human oversight, ensuring users stay informed and engaged.
Getting Clean Data and Avoiding Bias
Good data practices are essential to ensure fairness and accuracy in AI systems. A well-known example is Amazon’s 2015 hiring algorithm, which showed bias against resumes mentioning “women’s college” due to skewed historical data. To reduce bias, consider these strategies:
- Diverse Testing Groups: Microsoft’s Inclusive Design Toolkit involves people with varied abilities throughout the development process.
- Cultural Awareness: Google Translate adjusts language outputs to reflect cultural nuances.
- Ongoing Monitoring: Regular audits help catch and address biases in AI outputs early.
These steps not only improve fairness but also build confidence in AI-driven tools.
Using Emotion Detection Correctly
Emotion detection technology raises privacy concerns, with only 15% of Americans supporting its use in public ads. However, in the UK, 43.1% of young adults (18-24) are open to anonymized emotion data collection . To handle this technology responsibly, follow these guidelines:
Aspect | Best Practices |
---|---|
Privacy Protection | Clearly inform users about what data is collected |
User Control | Allow users to opt out of emotion detection features |
Transparency | Explain the limitations of emotion detection |
Bias Prevention | Involve diverse teams in the development process |
Data Usage | Collect only the data necessary for key features |
It’s worth noting that diversity in AI teams remains a challenge - 90% of Google’s AI research staff are men, and only 2.5% of full-time employees are Black. Emotion detection should be treated as a complementary tool, with human oversight remaining central in critical interactions. Following these practices can help ensure ethical and trustworthy AI applications.
Conclusion: Putting Users First in AI Design
AI thrives when designed with care and a focus on trust. Research from various countries highlights that trust in AI often depends on factors like education and professional experience. For example, only 19–21% of Australians find current AI safety measures satisfactory.
Transparency and Control
Being upfront about what AI can and can't do is key to earning trust. A great example is adjustable automation, which shows how clear communication can give users more control over their interactions with AI. Striking a balance between AI support and human decision-making is essential for greater acceptance.
Education and Accessibility
Making AI accessible to everyone is just as important. Microsoft's toolkit is a good example of how inclusive design can improve accessibility. Their Seeing AI app adjusts to individual needs, showing how clear communication can strengthen user trust.
To create AI systems people can trust, they must be lawful, ethical, and reliable. Users also need straightforward explanations of AI decisions to feel confident in the technology.
Here are some practical ways to put these principles into action:
Trust-Building Element | Implementation Strategy |
---|---|
Transparency | Offer layered, easy-to-understand explanations of AI decisions |
User Control | Let users adjust how much AI is involved in tasks |
Cultural Fluency | Design interfaces that consider diverse user backgrounds |
Error Recovery | Provide clear options for fixing mistakes and getting support |
Continuous Learning | Regularly gather user feedback and make improvements |