How AI Predicts Risks in Sprint Planning
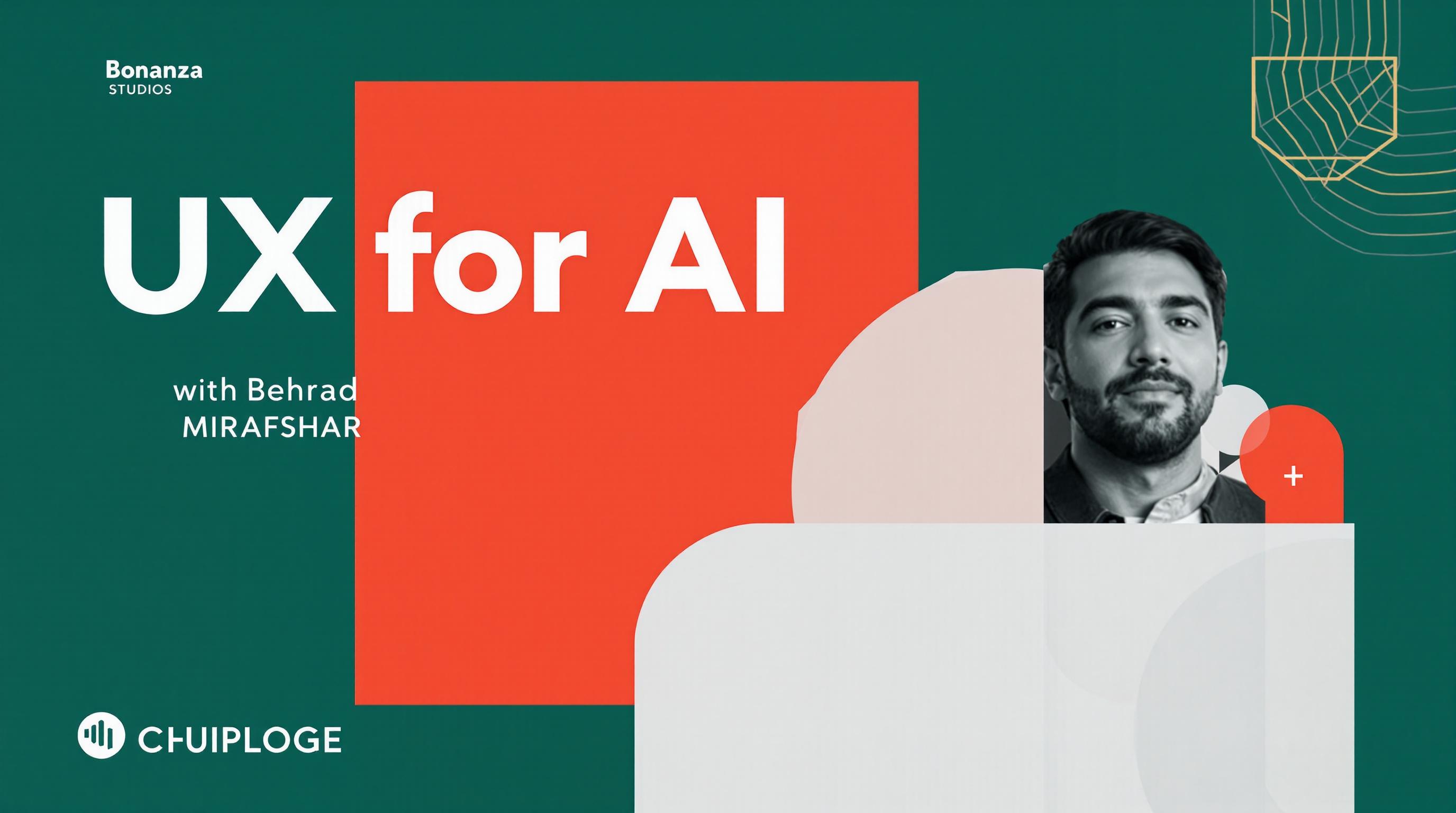
AI transforms sprint planning by predicting risks before they disrupt workflows. It analyzes historical data, team performance, and communication patterns to detect bottlenecks, balance workloads, and provide accurate timeline predictions. Here's what you need to know:
-
Key Risks Detected by AI:
- Timeline Risks: Predicts delays using sprint velocity and task completion rates.
- Resource Risks: Identifies gaps in team availability or skill sets.
- Communication Risks: Flags potential miscommunication through meeting participation and response times.
-
How AI Works:
- Uses machine learning to analyze past sprints.
- Employs natural language processing (NLP) to spot issues in team communication.
- Monitors real-time and historical data for early warnings.
-
Steps to Start Using AI:
- Test AI on small projects to measure its impact.
- Combine AI insights with human judgment for better decisions.
- Build team trust by showing how AI improves outcomes.
AI not only prevents risks but also optimizes team workflows, ensuring smoother sprints and better results. Ready to integrate AI into your sprint planning? Dive deeper into the details below.
AI: A New Thinking Partner in Agile Teams with Dan Neumann
AI Risk Detection in Sprint Planning
AI is reshaping how risks are identified and analyzed during sprint planning. By leveraging large volumes of historical data and performance metrics, AI delivers insights that help teams anticipate and address potential challenges. Below, we break down the key data sources and AI techniques that make this possible.
Key Data Sources for Identifying Risks
AI systems rely on several types of data to pinpoint potential sprint risks, including:
- Sprint Velocity Data: Historical trends in task completion rates and story point estimates.
- Team Performance Metrics: Patterns in productivity and capacity.
- Communication Patterns: Insights from collaboration tool usage, meeting attendance, and response times.
- Resource Allocation: Availability of team members and scheduling constraints.
At Bonanza Studios, we integrate these data streams into our AI-driven processes to generate detailed risk profiles for every sprint. This method has been instrumental in ensuring the success of our weekly design sprints and monthly delivery sprints.
AI Techniques for Risk Analysis
AI employs advanced methods to predict and assess sprint risks effectively.
Machine Learning Models
- Predictive algorithms analyze past sprint data to identify potential bottlenecks.
- Pattern recognition tools highlight recurring problems before they disrupt workflows.
- Natural Language Processing (NLP) scans team communications for signs of potential issues.
Risk Assessment Framework
AI systems categorize risks and analyze them using specific methods and indicators:
Risk Type | AI Analysis Method | Key Indicators |
---|---|---|
Timeline Risk | Prediction | Changes in sprint velocity and task completion rates |
Resource Risk | Capacity Check | Gaps in team availability and skill sets |
Communication Risk | Communication Check | Participation in meetings and response times |
Ultimately, the success of AI-driven risk detection depends on the quality and depth of historical data available.
Types of Risks AI Can Detect
Modern AI tools analyze both historical and real-time data to foresee potential sprint issues. These systems can identify risks that traditional sprint planning might miss. Below, we explore how AI addresses challenges related to resources, tasks, and communication.
Resource and Task Challenges
AI helps identify resource allocation issues by analyzing team capacity and workload patterns. Here's how it works:
- Matches required skills with available team expertise
- Compares assigned tasks to the team's past performance and velocity
- Spots scheduling conflicts and capacity issues by examining task dependencies
For instance, Bonanza Studios uses an AI-driven framework that leverages sprint velocity data to balance workloads and highlight bottlenecks early in the process.
Communication Challenges
AI can detect potential collaboration problems by monitoring key communication metrics, such as response times, meeting participation rates, and the consistency of shared documentation. By comparing these metrics to established norms, AI identifies early signs of miscommunication or misalignment. Addressing these gaps ensures smoother planning and reduces the risk of disruptions during sprints.
sbb-itb-e464e9c
Using AI Risk Insights
With AI-driven risk detection, teams can quickly tackle challenges as they arise.
Fixing High-Priority Issues
AI tools help identify and rank risks based on how much they could impact sprint success. By sorting risks by severity and urgency, teams can focus on solving the most pressing problems first.
Here’s how to handle high-priority risks:
- Review key tasks that could delay progress.
- Fill knowledge gaps through training or by reallocating team members.
- Resolve conflicts caused by overlapping resource needs.
After addressing these major risks, the next step is ensuring workloads are evenly distributed across the team.
Balancing Team Workload
Keep an eye on factors like task complexity, work pace, and team capacity. Adjust workloads as needed to maintain steady progress throughout the sprint. This approach complements earlier risk detection efforts and helps prevent bottlenecks.
Monitoring Sprints with AI
AI insights feed directly into sprint management tools, helping teams make informed decisions during the sprint. Continuous tracking evaluates performance and ensures any adjustments to risks or resources are effective.
Monitoring Focus | AI Insights Provided | Action Items |
---|---|---|
Velocity Trends | Daily progress vs. sprint goals | Adjust daily workload |
Risk Indicators | Early warning signals | Take quick corrective action |
Resource Usage | Team capacity utilization | Reassign tasks as needed |
To get the most out of AI monitoring:
- Set up custom alerts for risk thresholds.
- Review daily AI-generated insights.
- Measure how effective your solutions are over time.
This structured approach keeps sprints on track, ensures workloads stay manageable, and addresses risks promptly.
Steps to Add AI to Sprint Planning
Testing AI on Small Projects
Start by using AI for risk prediction on smaller sprints to measure its impact. Pick a pilot project with clear success criteria to assess how AI performs. Focus on identifying straightforward risk indicators like task dependencies and resource usage. Key metrics to track include:
- Accuracy of risk predictions
- Time saved in identifying risks
- Reduced sprint disruptions
- Increases in team productivity
After proving its value in smaller projects, combine AI insights with human expertise for broader implementation.
AI and Human Decision-Making
Blend AI's analytical strengths with human judgment. AI is excellent at spotting patterns and analyzing data, but human experience is essential for understanding context and nuances.
Decision Area | AI's Role | Human's Role |
---|---|---|
Risk Detection | Spot patterns and anomalies | Assess context and team dynamics |
Resource Planning | Suggest optimal allocations | Factor in individual strengths |
Timeline Estimates | Provide data-driven predictions | Adjust for team-specific realities |
Priority Setting | Highlight high-risk items | Make strategic trade-offs |
This balance ensures effective decision-making while leveraging both AI and human strengths.
Building Team Trust in AI
Encourage team buy-in by prioritizing transparency and open communication. Steps to build trust include:
- Sharing success metrics to show how AI improves sprint outcomes.
- Offering training so team members understand how AI generates its recommendations.
- Keeping AI processes visible, ensuring the team knows how decisions are made.
Set up feedback loops to track AI's accuracy and gather suggestions for improvement. This collaborative method not only improves the system but also boosts team confidence in its use.
Bonanza Studios Solutions
Bonanza Studios takes AI risk insights and applies them to improve sprint planning with precise, practical solutions. Their framework blends data-driven insights with human expertise to fine-tune sprint processes.
The studio's AI-powered framework evaluates sprint data across three key areas:
Risk Analysis Dimension | AI Capabilities | Team Benefits |
---|---|---|
Sprint Performance | Predictive analytics for resource allocation | Better workload management and improved delivery timelines |
Task Dependencies | Automated mapping and conflict detection | Fewer bottlenecks and smoother workflows |
Team Dynamics | Analysis of communication patterns | Stronger collaboration and increased productivity |
Bonanza Studios combines the skills of AI technologists and product strategists to help clients implement risk-aware sprint planning. Their methods have delivered measurable results, as highlighted by client feedback:
"Bonanza has surpassed all our expectations. We regard them as our Chief Growth & Product Officer." - Ahswant Akula, CEO & Co-founder
Their risk management process includes:
- AI-Driven UX Design: Adjusts workflows in real-time based on risk data
- Quick Deployment: Integrates AI risk detection tools into existing sprint systems
- Data-Based Adjustments: Continuously refines sprint parameters using AI insights
For businesses moving toward AI-integrated sprint planning, Bonanza Studios offers full support through their digital transformation services. They focus on building modern digital infrastructure, ensuring a solid foundation for managing risks.
With a strong focus on human-centric design, their AI predictions are tailored to be actionable and aligned with team strengths. Their cross-functional team adapts sprint plans quickly to address risks as they arise.
Conclusion
AI-driven risk detection has transformed sprint planning from merely solving problems after they arise to preventing risks before they happen. With AI's ability to analyze data, teams can now pinpoint and tackle potential obstacles early, ensuring smoother project timelines and deliverables.
Here are three main benefits of using AI in sprint planning:
- Accuracy: AI uses historical and real-time data to predict disruptions with precision.
- Quick Action: Teams can address risks immediately as they surface.
- Ongoing Improvement: Prediction models get better with every sprint.
These advantages make AI an essential tool for enhancing team strategies. When AI insights are paired with human judgment, teams achieve better results.
To make the most of AI for risk prevention, organizations should:
- Assemble teams that combine AI expertise with other disciplines.
- Keep agile practices at the core of their processes.
- Regularly review and fine-tune AI risk detection settings.
As sprint planning evolves, AI's role in predicting risks will only grow more important, helping teams manage complex projects while delivering consistent results. This forward-thinking approach strengthens risk management and prepares teams to handle future challenges effectively.